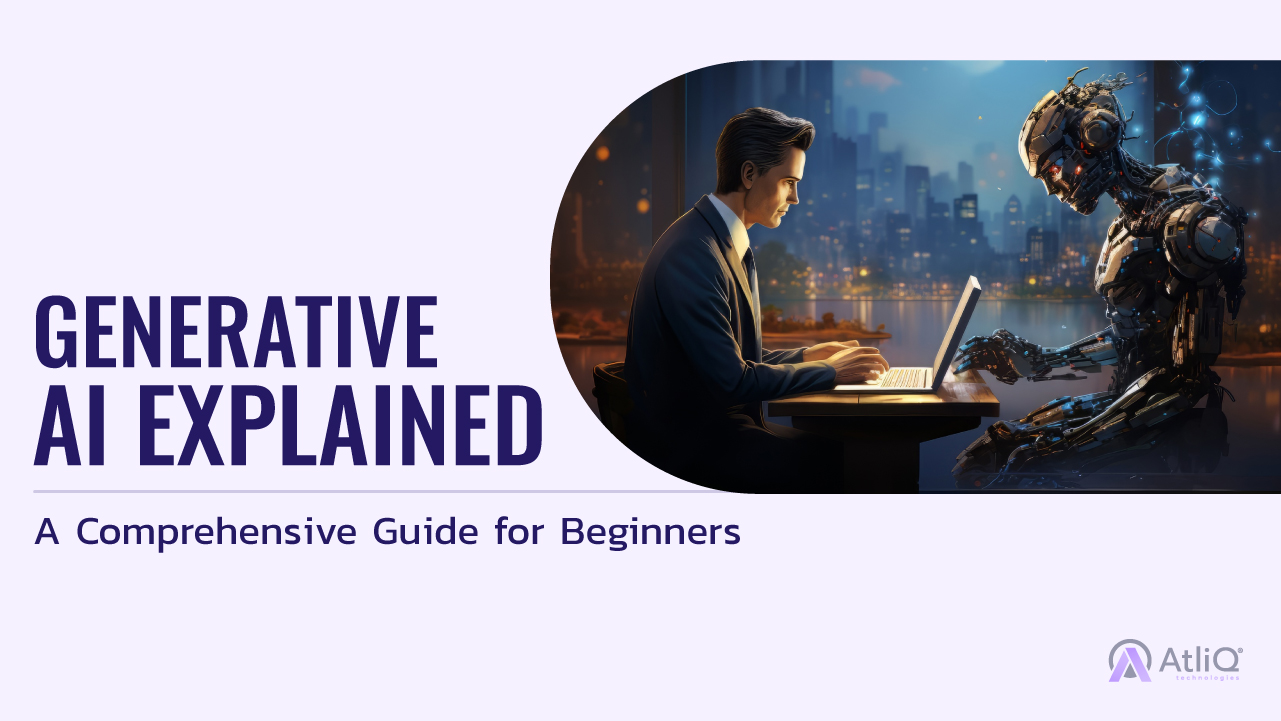
Imagine a world where machines can create art, compose music, and write stories that rival the works of human masters. A world where artificial intelligence (AI) can generate realistic images, videos, and even entire virtual worlds from scratch. Welcome to the fascinating realm of Generative AI, a groundbreaking technology that is revolutionizing the way we create, innovate, and perceive the boundaries of what’s possible. In recent years, Generative AI has emerged as one of the most exciting and rapidly advancing fields in artificial intelligence. From generating photorealistic images of non-existent people to creating music that sounds like it was composed by famous musicians, Generative AI is pushing the limits of creativity and imagination.
But what is Generative AI, and how does it work? What are its potential applications, and what challenges and considerations come with this powerful technology? Whether you’re a tech enthusiast, an artist, a researcher, or simply someone curious about the latest advancements in AI, this blog post will provide you with a comprehensive understanding of Generative AI and its potential to transform various industries.
Definition of Generative AI: Generative AI is a subset of artificial intelligence that focuses on creating new content, such as images, videos, music, or text, using machine learning algorithms. Unlike traditional AI systems designed to recognize patterns or make predictions based on existing data, Generative AI aims to generate novel and original content that resembles the data it was trained on.
Generative AI models learn from vast amounts of data, such as images, videos, or text, to understand the underlying patterns and structures. By capturing the essence of this data, these models can generate new content that shares similar characteristics or style to the original data, yet is unique.
How does Generative AI work?
At the core of Generative AI are deep learning algorithms, specifically neural networks, designed to learn from data in an unsupervised or semi-supervised manner. The two most prominent approaches to Generative AI are Generative Adversarial Networks (GANs) and Variational Autoencoders (VAEs).
Generative Adversarial Networks (GANs): GANs consist of two neural networks – a generator and a discriminator – that work together competitively. The generator creates new content, while the discriminator evaluates the generated content and provides feedback on how realistic it is compared to real data. Through this iterative process, the generator learns to create content that is increasingly indistinguishable from real data, while the discriminator becomes better at spotting the differences between real and generated content.
Variational Autoencoders (VAEs): VAEs are another popular approach to Generative AI. They consist of an encoder network that compresses input data into a lower-dimensional latent space and a decoder network that reconstructs the original data from the latent representation. By sampling from the latent space and passing it through the decoder, VAEs can generate new content that shares similar characteristics with the original data.
Both GANs and VAEs learn to capture the statistical properties of the training data, enabling them to generate new content that follows the same distribution. As these models are trained on larger and more diverse datasets, they can create increasingly sophisticated and realistic content.
Generative AI models can also be conditioned on specific inputs, such as text descriptions or class labels, to generate content that meets certain criteria. This has led to the development of models like GPT-3 for text generation and DALL-E for image generation based on textual prompts. As Generative AI continues to advance, we can expect to see even more impressive and diverse applications across various domains, from creative industries to healthcare and beyond.
Popular Generative AI Tools:
In recent years, various Generative AI tools have emerged, making it easier for users to experiment with and leverage the power of generative models. These tools offer a wide range of capabilities, from text and image generation to music composition and video synthesis. Here are some of the most popular Generative AI tools:
- OpenAI GPT-3: GPT-3 is a powerful language model that can generate human-like text, answer questions, and even write code. It has been used for a variety of applications, including chatbots, content creation, and programming assistants.
- DALL-E & DALL-E 2: Developed by OpenAI, DALL-E and its successor DALL-E 2 are models that can generate images from textual descriptions. These tools have showcased impressive results, creating highly realistic and creative images based on user prompts.
- Midjourney: Midjourney is an AI-powered image generation tool that allows users to create stunning visual art using simple text commands. It has gained popularity among artists and designers for its ability to generate unique and imaginative images.
- Stable Diffusion: Stable Diffusion is an open-source image generation model that can produce high-quality images from text descriptions. It has democratized access to generative image modeling and has been widely used by the creative community.
- Jukebox: Developed by OpenAI, Jukebox is a generative model for music that can create new songs in various genres and styles. It can generate music that mimics the style of popular artists and can even create novel musical compositions.
- Runway ML: Runway ML is a platform that offers a suite of Generative AI tools for creators, including models for image and video editing, style transfer, and text-to-image generation. It provides a user-friendly interface for individuals to experiment with generative models without requiring deep technical knowledge.
These are just a few examples of the many Generative AI tools available. As the field continues to advance, we can expect to see even more powerful and accessible tools that empower users to harness the potential of generative models for a wide range of creative and practical applications.
Challenges and Ethical Considerations For Generative AI
- Deepfakes & Misinformation: Generative AI has the potential to create highly realistic fake content, known as deepfakes. These can be used to spread misinformation, manipulate public opinion, or even harass individuals. Deepfakes can be in the form of generated images, videos, or text that are difficult to distinguish from real content. This poses significant challenges in combating the spread of fake news and maintaining trust in digital media.
- Privacy & Data Security: Generative AI models require vast amounts of training data, which can include personal and sensitive information. The collection, storage, and use of this data raise concerns about privacy and data security. There is a risk of data breaches or misuse of personal information if proper safeguards are not in place. Additionally, the generated content itself may contain traces of the training data, potentially revealing sensitive details.
- Legal & Regulatory Issues: The rapid advancements in Generative AI have outpaced the development of legal and regulatory frameworks. There are questions about intellectual property rights, copyright, and ownership of generated content. For example, if an AI model is trained on copyrighted material and generates similar content, it raises concerns about infringement and fair use. Additionally, there are challenges in attributing responsibility and liability for the actions or outputs of Generative AI systems.
- Bias & fairness In Generated Content: Generative AI models can inherit and amplify biases present in the training data. If the data used to train these models contains historical biases or underrepresentation of certain groups, the generated content may perpetuate these biases. This can lead to unfair or discriminatory outcomes, particularly in applications such as hiring, lending, or criminal justice. Ensuring fairness and mitigating bias in Generative AI is an ongoing challenge that requires careful data curation, model design, and evaluation.
- Computational Resources & Environmental Impact: Training and deploying large-scale Generative AI models require significant computational resources and energy consumption. The environmental impact of these models, particularly in terms of carbon footprint, is a growing concern. The high energy demands of AI systems contribute to greenhouse gas emissions and can have negative consequences for sustainability. Researchers and organizations are exploring ways to develop more efficient models and reduce the environmental impact of Generative AI.
Addressing these challenges and considerations is crucial for the responsible development and deployment of Generative AI. It requires ongoing research, collaboration between stakeholders, and the establishment of ethical guidelines and regulatory frameworks. By proactively addressing these issues, we can harness the benefits of Generative AI while mitigating potential risks and negative consequences.
Future of Generative AI
ADVANCEMENTS & TRENDS IN GENERATIVE AI RESEARCH: Generative AI is a rapidly evolving field, with new breakthroughs and advancements emerging at a remarkable pace. One of the key trends in Generative AI research is the development of more efficient and scalable models. Researchers are exploring techniques such as transfer learning, meta-learning, and few-shot learning to enable models to learn from smaller amounts of data and generalize to new tasks more effectively.
Another important trend is the integration of Generative AI with other AI techniques, such as reinforcement learning and multi-modal learning. This allows for the creation of more sophisticated and interactive generative models that can learn from and respond to user feedback in real-time. Additionally, there is a growing interest in developing explainable and interpretable Generative AI models, which can provide insights into their decision-making process and enhance trust in their outputs.
POTENTIAL IMPACT ON VARIOUS INDUSTRIES: Generative AI has the potential to revolutionize various industries and transform the way we create and consume content. In the creative industries, such as art, music, and design, Generative AI can assist and augment human creativity by providing new ideas, inspiration, and tools for content creation. It can also enable the personalization and customization of content based on individual preferences and needs.
OPPORTUNITIES FOR INNOVATION & COLLABORATION: The future of Generative AI presents immense opportunities for innovation and collaboration across different domains. As the technology advances, there will be a growing need for interdisciplinary collaboration between AI researchers, domain experts, artists, and policymakers to ensure the responsible and beneficial development of Generative AI.
There are opportunities for startups and companies to develop new products and services powered by Generative AI, catering to various industries and user needs. Collaboration between academia and industry can foster the transfer of research breakthroughs into real-world applications and drive further advancements in the field.
Moreover, Generative AI can enable new forms of creative expression and collaboration between humans and machines. Artists, musicians, and writers can leverage Generative AI tools to explore new creative possibilities and push the boundaries of their respective fields. Collaborative platforms and communities can emerge, fostering the exchange of ideas, techniques, and best practices in Generative AI.
As we have seen, Generative AI is not without its challenges and considerations. Ethical concerns, such as deepfakes and data privacy, legal and regulatory issues, bias and fairness, and the environmental impact of computational resources, are all critical aspects that need to be addressed for the responsible development and deployment of Generative AI. Despite these challenges, the future of Generative AI is incredibly promising. With ongoing advancements in research, the integration of Generative AI with other AI techniques, and the increasing availability of tools and platforms, we can expect to see a surge of innovation and collaboration in this field. The potential impact of Generative AI on various industries is immense, and it is up to researchers, developers, policymakers, and users to work together to harness its benefits while mitigating potential risks.
As a leading provider of data analytics and AI solutions, AtliQ Technologies is at the forefront of helping organizations leverage the power of Generative AI. Our team of experienced data scientists and AI experts can assist you in incorporating Generative AI into your projects, from ideation and development to deployment and evaluation. Whether you are looking to enhance your creative processes, optimize your business operations, or develop new products and services, AtliQ Technologies can provide the expertise and support you need to succeed.
By partnering with AtliQ Technologies, you can stay ahead of the curve and capitalize on the opportunities presented by Generative AI. Our solutions are tailored to your specific needs and goals, ensuring that you can harness the full potential of this transformative technology. With our help, you can navigate the challenges, mitigate risks, and unlock new possibilities for innovation and growth.
So, if you are ready to embark on your Generative AI journey, contact AtliQ Technologies today. Let us help you stay at the forefront of this exciting field and drive your organization towards a more innovative, creative, and successful future.