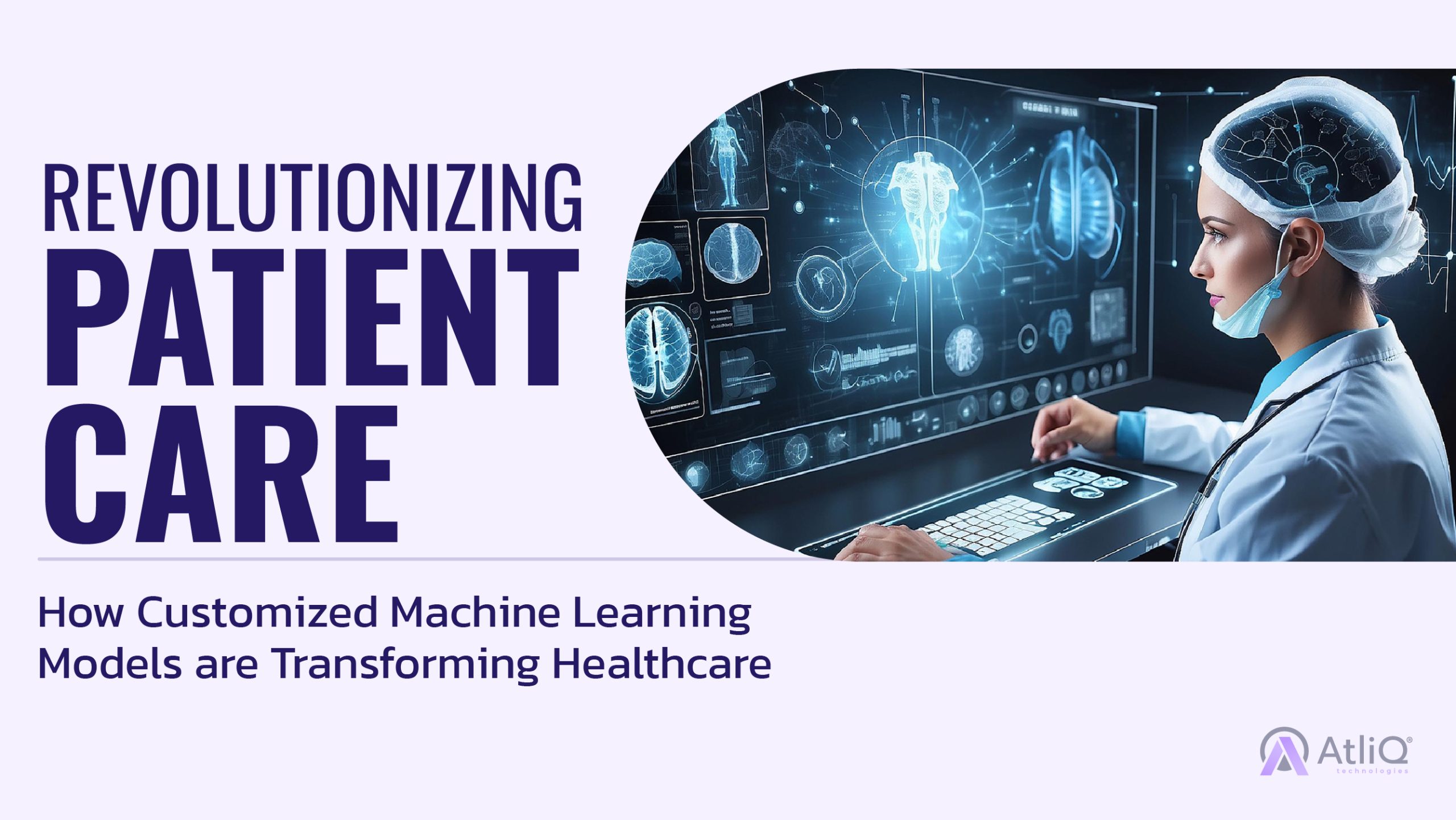
Imagine a future where your healthcare provider has access to a sophisticated ML model that is tailored specifically to you. This model would be trained on a vast array of data points, including your medical history, genetic profile, and real-time health metrics. It could predict potential health risks, recommend personalized treatment plans, and monitor your progress with unparalleled precision.
Now, stop imagining, because it is the reality already beginning to take shape. The customization of ML models for patient care is poised to revolutionize the healthcare industry, offering a new paradigm of personalized medicine that puts the individual at the center of the equation. In this blog post, we will delve into the exciting world of customized ML models, exploring their applications, challenges, and the incredible potential they hold for transforming patient care as we know it.
The Importance of Technology in Healthcare
In recent years, technology has become an integral part of the healthcare industry. From electronic health records (EHRs) to telemedicine, technological advancements have transformed the way healthcare is delivered, making it more efficient, accessible, and patient-centric. The integration of technology has not only improved the quality of care but has also reduced costs and enhanced patient outcomes.
One of the most significant technological breakthroughs in healthcare has been the adoption of EHRs. These digital records have replaced traditional paper-based systems, allowing healthcare providers to store, access, and share patient information seamlessly. EHRs have streamlined workflows, reduced medical errors, and facilitated better communication among healthcare professionals, ultimately leading to improved patient care.
Moreover, telemedicine has emerged as a game-changer in healthcare delivery. With the help of video conferencing and remote monitoring tools, patients can now consult with their healthcare providers from the comfort of their own homes. Telemedicine has proven particularly valuable in rural and underserved areas, where access to healthcare services may be limited. It has also played a crucial role during the COVID-19 pandemic, enabling patients to receive care while minimizing the risk of exposure to the virus.
Introducing Machine Learning (ML) in Healthcare
Among the various technological advancements in healthcare, machine learning (ML) has emerged as a powerful tool with immense potential. ML is a subset of artificial intelligence (AI) that focuses on the development of algorithms and models that can learn from data and improve their performance over time without being explicitly programmed. One of the key advantages of ML in healthcare is its ability to process and analyze large volumes of complex data quickly and accurately. This is particularly important in the era of big data, where the amount of medical information generated is growing exponentially. ML algorithms can sift through this data, identifying relevant insights and patterns that may be missed by human observers, thereby enhancing the accuracy and speed of diagnosis and treatment.
Moreover, ML has the potential to enable personalized medicine, where treatment plans are tailored to the unique characteristics and needs of individual patients. By analyzing a patient’s medical history, genetic profile, and lifestyle factors, ML models can predict the likelihood of certain diseases, recommend optimal treatment options, and monitor treatment response in real time.
Applications of Customized ML Models in Patient Care
Diagnosis and Disease Prediction
One of the most significant benefits of machine learning in healthcare is its ability to revolutionize the way diseases are diagnosed and predicted. Customized ML models can analyze vast amounts of patient data, including medical records, imaging scans, and genetic information, to identify patterns and risk factors associated with specific diseases. This enables earlier detection of diseases, even before symptoms appear, allowing for timely intervention and improved patient outcomes.
Moreover, customized ML models can enhance the accuracy of diagnosis by considering a wide range of factors specific to each patient. By training on diverse datasets and incorporating patient-specific features, these models can outperform traditional diagnostic methods, reducing the likelihood of misdiagnosis and ensuring that patients receive appropriate treatment.
- Early Detection of Diseases: Customized ML models can be trained to recognize subtle changes in patient data that may indicate the onset of a disease. For example, ML algorithms can analyze medical imaging scans to detect early signs of cancer, enabling prompt treatment and improving survival rates. Similarly, ML models can identify risk factors for chronic conditions such as diabetes or cardiovascular disease, allowing for proactive interventions to prevent or delay the onset of these conditions.
- Improved Accuracy in Diagnosis: Traditional diagnostic methods often rely on a limited set of symptoms and test results, which can lead to inaccurate or delayed diagnoses. Customized ML models, on the other hand, can consider a much broader range of factors, including a patient’s medical history, lifestyle habits, and genetic profile. By analyzing these diverse data points, ML models can provide more accurate and personalized diagnoses, reducing the risk of misdiagnosis and ensuring that patients receive the most appropriate treatment.
Treatment Planning and Optimization
Another key application of customized ML models in patient care is treatment planning and optimization. By leveraging machine learning for patient care, healthcare providers can develop personalized treatment plans that are tailored to the unique needs and characteristics of each patient.
- Personalized Treatment Plans: Customized ML models can analyze a patient’s data to identify the most suitable treatment options based on their specific condition and individual characteristics. For example, ML algorithms can help determine the optimal medication dosage, taking into account a patient’s age, weight, and liver function. This personalized approach can maximize treatment efficacy while minimizing the risk of adverse side effects.
- Predicting Treatment Response: ML models can also be used to predict a patient’s response to specific treatments, allowing healthcare providers to make informed decisions about the most appropriate course of action. By analyzing data from previous patients with similar characteristics and treatment histories, ML algorithms can identify patterns and predict the likelihood of a positive response to a particular intervention. This can help avoid unnecessary or ineffective treatments, reducing healthcare costs and improving patient outcomes.
Patient Monitoring and Management
Customized ML models also have significant potential in the realm of patient monitoring and management, particularly for individuals with chronic conditions. By leveraging remote monitoring technologies and ML algorithms, healthcare providers can keep track of patient’s health status in real time, enabling early intervention and prevention of complications.
- Remote Monitoring of Chronic Conditions: ML models can be trained to analyze data from wearable devices and remote monitoring systems, such as smartphones or smartwatches, to track patients’ vital signs, activity levels, and medication adherence. This continuous monitoring allows healthcare providers to detect deviations from normal patterns and intervene promptly to prevent the worsening of chronic conditions.
- Early Intervention and Prevention of Complications: By constantly analyzing patient data and identifying potential risk factors, customized ML models can enable early intervention and prevention of complications. For example, ML algorithms can detect subtle changes in a patient’s glucose levels, alerting healthcare providers to the need for adjustments in insulin dosage or lifestyle modifications to prevent the development of diabetic complications.
While the benefits of machine learning in healthcare are numerous, it is essential to acknowledge the potential disadvantages. One of the main challenges is the need for large, diverse, and high-quality datasets to train accurate and unbiased ML models. Additionally, there are concerns regarding data privacy and security, as well as the potential for algorithmic bias if models are trained on datasets that are not representative of the broader population. Despite these challenges, the application of customized ML models in patient care holds immense promise for transforming healthcare delivery. By harnessing the power of machine learning for patient care, we can move towards a future where healthcare is truly personalized, proactive, and data-driven, ultimately improving patient outcomes and quality of life.
Success Stories Of Customized ML Models In Healthcare
Personalized Cancer Treatment at Memorial Sloan Kettering Cancer Center (MSKCC): MSKCC has developed a machine learning model called “Watson for Oncology” in collaboration with IBM. This customized ML model analyzes vast amounts of patient data, including medical records, genetic information, and treatment histories, to provide personalized cancer treatment recommendations. The model takes into account the unique characteristics of each patient, such as their age, gender, and specific type of cancer, to suggest the most effective treatment options. This approach has led to improved patient outcomes and reduced side effects associated with cancer treatment.
Predictive Analytics for Chronic Disease Management at Kaiser Permanente: Kaiser Permanente, a large integrated healthcare system in the United States, has implemented customized ML models to improve the management of chronic diseases such as diabetes and heart failure. By analyzing patient data from electronic health records, these models can predict the likelihood of complications and hospital readmissions, enabling proactive interventions and personalized care plans. For example, the ML model can identify patients at high risk of developing diabetic foot ulcers and alert healthcare providers to initiate preventive measures, such as regular foot exams and patient education.
Early Detection of Sepsis at Johns Hopkins Hospital: Researchers at Johns Hopkins Hospital have developed a customized ML model to predict the onset of sepsis, a life-threatening condition caused by the body’s response to infection. The model analyzes real-time patient data, including vital signs, laboratory results, and electronic health records, to identify patients at risk of developing sepsis. By providing early warnings, the model enables healthcare providers to initiate timely interventions, such as administering antibiotics and supportive care, which can significantly improve patient outcomes and reduce mortality rates.
Personalized Mental Health Treatment at McLean Hospital: McLean Hospital, a leading psychiatric institution, has partnered with Mindstrong Health to develop customized ML models for mental health treatment. These models analyze data from patients’ smartphones, such as their typing patterns, screen time, and location data, to identify early signs of mental health deterioration. By detecting changes in behavior and mood, the models can alert healthcare providers to intervene promptly and adjust treatment plans accordingly. This personalized approach has shown promise in improving outcomes for patients with conditions such as depression, bipolar disorder, and schizophrenia.
These real-world examples demonstrate the transformative potential of customizing ML models for patient care. By leveraging the power of machine learning and personalized data analysis, healthcare organizations can improve the accuracy of diagnoses, optimize treatment plans, and enhance patient monitoring and management. As more healthcare institutions adopt these innovative approaches, we can expect to see significant advancements in patient outcomes, quality of care, and overall healthcare efficiency.
The customization of machine learning models for patient care represents a significant milestone in the evolution of healthcare. By harnessing the power of data and advanced algorithms, we can unlock new possibilities for personalized medicine, improved patient outcomes, and enhanced healthcare efficiency.
Embrace the power of customized machine learning models and join the revolution in personalized patient care today.